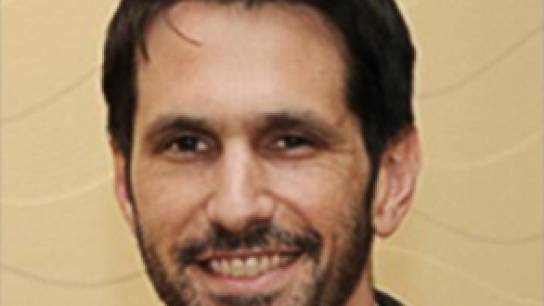
Dr Stephanus Malherbe, Evaluate4mTB Study Lead
Evaluate4mTB, a sub-study of PredictTB, is developing and testing a new machine learning algorithm to automate the analysis of tuberculosis patient scans. Led by Dr Stephanus Malherbe from Stellenbosch University, Evaluate4mTB aims to complement its parent study by providing additional analyses of scans from all PredictTB’s 516 participants, focusing on scans at month four and six of treatment.
Evaluate4mTB is run as a sub-study of the PredictTB trial and led by Dr Stephanus Malherbe, who, in 2018, was awarded a Career Development Fellowship by the second European and Developing Countries Clinical Trial Programme (EDCTP2) for the study. Capacity development is a core EDCTP activity, and the Career Development Fellowships aim to support early and mid-career scientists to develop their clinical research skills, providing an opportunity for talented scientists to establish themselves as independent researchers and team leaders.
The PredictTB study's rationale is to use data from scans, assets, and culture to determine which patients are eligible for treatment shorter than the current standard of care. It also aims to demonstrate that the 18-months treatment success rate of standard treatment stopped after four months is not inferior to treatment stopped after six months.
In the frame of the Evaluate4mTB study, Dr Malherbe and his team perform additional analyses of PET/CT scans from PredictTB's patients at month four and six of treatment. Evaluate4mTB complements the parent study by comparing scan metrics between outcome groups and treatment arms in order to gain insights into how particular biomarkers relate to patients' responses to different treatment durations. According to Dr Malherbe, this should facilitate the discovery of biomarkers to evaluate the end-of-treatment status of the TB infection in the lung, regardless of treatment duration.
Evualute4mTB aims to set a frame for what criteria patients should meet during specific treatment durations to be considered eligible for shorter treatment. Determining a reliable threshold at any time during treatment would be a major step towards easy and affordable tests to allow individualized treatment and precision medicine.
Dr Malherbe and his team will study scans from all PredictTB's 516 participants. Manually analyzing scans from hundreds of patients is a time-consuming job. By applying machine learning to the process, Dr Malherbe hopes to be able to automate much of the work.
Over the past years, he has been collaborating with a team of biomedical engineers and nuclear physicians at Stellenbosch University to develop software that can study and analyze digital lung scans. The automated analysis method's technology is based on previously existing models that the Stellenbosch researchers have improved and adjusted for their novel use-case.
In the first step, Dr Malherbe used semi-automated analysis to go over 300 scans. With this method, the software analyzed each voxel in the lungs to determine which scans showed normal vs abnormal lung functions. Dr Malherbe was, however, still drawing the lung volumes for the scans.
Manual lung segmentation for analysis is already ongoing for the main PredictTB study. However, for the next batch of 1,800 scans that Evaluate4mTB should analyze, Dr Malherbe will test a new fully automated analysis method. The two biomedical engineers on his team have written and trained a machine-learning algorithm to draw lung volumes. Dr Malherbe will monitor the algorithm's work carefully and perform randomized manual quality-checks of their scan analyses.
Dr Malherbe is pleased with the progress of his study to date. He believes that he and his team are in a good position to reach their scientific goals, despite that the study’s initial timeline had to be adjusted after delays caused by the coronavirus lockdown in South Africa earlier this year.
Suppose the automated analysis model works as planned, Evaluate4mTB's algorithms will help speed up scan analyses in clinical trials and provide important directions for how PET/CT scans should be used in TB research.